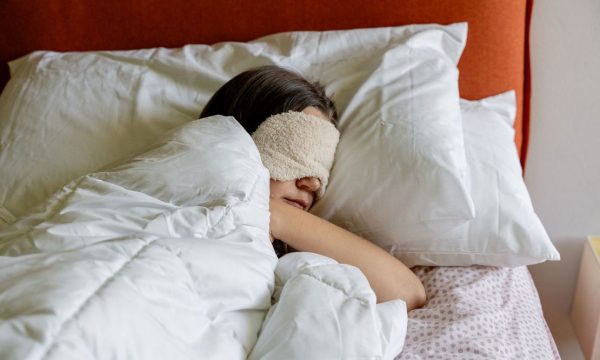
United States: Sleep tracking devices may offer insights that reach far beyond nightly rest patterns; they could provide vital clues about chronic health conditions, including diabetes and sleep apnea, as well as infectious diseases like COVID-19. This remarkable revelation is part of a study analyzing an expansive dataset of five million recorded nights across nearly 33,000 individuals. From this analysis, researchers identified five distinct sleep “phenotypes” or types, which can be further delineated into 13 nuanced subtypes.
Crucially, the frequency and manner by which individuals transition between these sleep phenotypes potentially contain two to ten times more diagnostic information than relying solely on a person’s primary sleep type. This transformative study was published on June 20, 2024, in npj Digital Medicine.
Unlocking Patterns with the Oura Ring
Utilizing data from the Oura Ring—a sophisticated wearable that monitors sleep, skin temperature, and other biometric signals—researchers analyzed individuals over several months, documenting instances of chronic ailments like diabetes, sleep apnea, and infections, including COVID-19 and the flu. These findings revealed dynamic shifts in sleep phenotypes that aligned with changing health conditions, painting a data-driven “map” of sleep transitions, according to technologynetworks.com.
“We observed that subtle variations in sleep quality highlighted health risks that wouldn’t necessarily emerge through average sleep metrics or standard health questionnaires, showcasing the profound potential of wearables to unearth overlooked risks,” explained Benjamin Smarr, one of the study’s senior contributors and a faculty member at the Jacobs School of Engineering and Halicioglu Data Science Institute, UC San Diego.
Phenotypes as Windows into Health Risks
Examining extensive sleep data allowed researchers to isolate five principal sleep phenotypes:
– Phenotype 1: Consistent, uninterrupted sleep for approximately eight hours nightly, mirroring the NIH’s recommended rest duration and representing the most prevalent sleep pattern.
– Phenotype 2: Alternating between full-night sleep and fragmented rest, often limited to short intervals of less than three hours.
– Phenotype 3: Predominantly uninterrupted sleep, with occasional disruptions, approximately one night per week. These interruptions generally involve one extended five-hour rest period and a shorter three-hour stint, according to technologynetworks.com.
– Phenotype 4: Mainly continuous sleep interspersed with rare interruptions, in which long sleep bouts are punctuated by a brief period of mid-sleep waking.
– Phenotype 5: Highly disrupted sleep every night, with extremely brief rest periods—a rare but revealing phenotype indicative of intense sleep disturbances.
Tracing Sleep Types Over Time
To capture how sleep phenotypes evolved, lead researcher Varun Viswanath designed a spatial model covering all five million nights, where each phenotype was visualized as distinct “islands” composed of similar weekly sleep patterns. This approach allowed researchers to identify patterns in individuals’ “journeys” between these islands.
Significantly, it was the frequency of switching between these sleep phenotypes—not the baseline phenotype—that often distinguished individuals with chronic conditions like diabetes and sleep apnea. In this data-driven “sleep landscape,” occasional yet consistent switching between phenotypes provided essential health information.
In tracing these routes, researchers observed that while most people experienced intermittent periods of disrupted sleep, those moments of transition held crucial insights. “Minor variations in sleep disruptions are highly informative, even when infrequent,” noted Professor Edward Wang, coauthor and faculty member in Electrical and Computer Engineering at UC San Diego. “This points not only to sleep quality but also to the hidden health indicators embedded within our sleep patterns,” as per technologynetworks.com.
Interestingly, individuals with fragmented sleep patterns tended to shift away from disrupted sleep over time, but the frequency of visits to these disrupted patterns revealed a great deal about their overall health.
“If we picture sleep as a landscape, it’s less about where someone resides on that map and more about how often they leave their usual area,” remarked Viswanath, the study’s corresponding author.
Broader Implications for Public Health
This study, rooted in the TemPredict dataset—data gathered by the University of California, San Francisco during the 2020 COVID-19 pandemic—hints at the broader public health implications of monitoring sleep patterns on a large scale. Tracking these trends could reveal early indicators of chronic disease susceptibility or vulnerability to infections, allowing for timely public health interventions, according to technologynetworks.com.
This pioneering work, led by Smarr, Wang, and Professor Ashley E. Mason of UCSF, underscores the potential of wearable technology not only to enrich our understanding of sleep but also to inform a new paradigm in health monitoring.